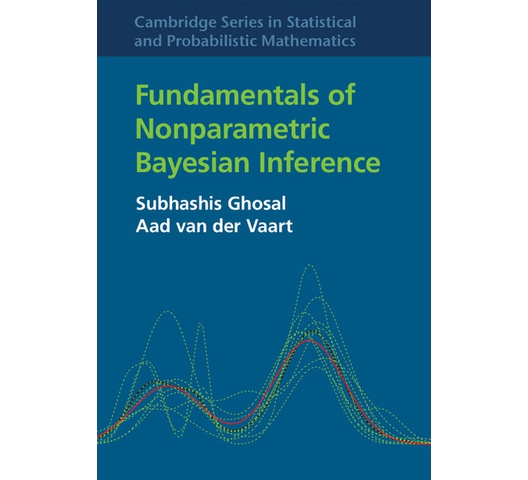
Fundamentals of Nonparametric Bayesian Inference
Explosive growth in computing power has made Bayesian methods for infinite-dimensional models - Bayesian nonparametrics - a nearly universal framework for inference, finding practical use in numerous subject areas. Written by leading researchers, this authoritative text draws on theoretical advances of the past twenty years to synthesize all aspects of Bayesian nonparametrics, from prior construction to computation and large sample behavior of posteriors. Because understanding the behavior of posteriors is critical to selecting priors that work, the large sample theory is developed systematically, illustrated by various examples of model and prior combinations. Precise sufficient conditions are given, with complete proofs, that ensure desirable posterior properties and behavior. Each chapter ends with historical notes and numerous exercises to deepen and consolidate the reader's understanding, making the book valuable for both graduate students and researchers in statistics and machine learning, as well as in application areas such as econometrics and biostatistics.
KES 15,821
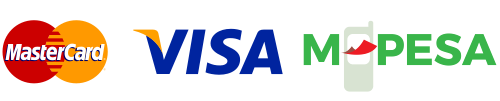
International delivery
Free click & collect
UPC | 9781108206075 |
---|---|
Author | Subhashis Ghosal, Aad van der Vaart |
Pages | 0 |
Language | English |
Format | EPUB |
Publisher | Cambridge University Press |
SKU | 9781108206075 |
None